AI and Psychedelics: Transforming Healthcare Through Innovation
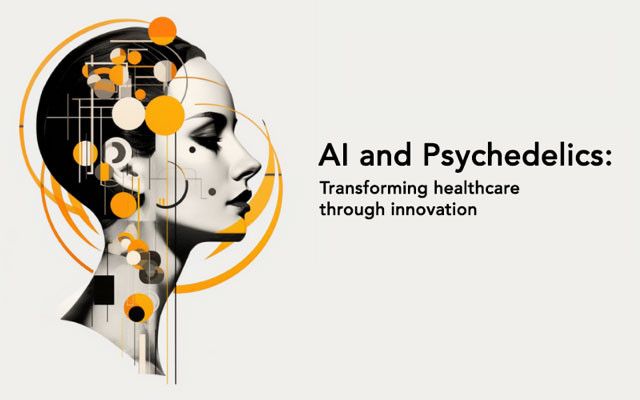
Artificial intelligence (AI) is defined as the ability of technology to stimulate intelligent behaviour and critical thinking that can be considered comparable to that seen in a human being. AI systems can recognise patterns, learn from experience, and make analytical decisions. Machine learning, a common form of AI, can identify and analyse patterns within data and it is starting to be used in psychedelic research. Psychedelics have shown therapeutic potential in clinical trials for mental illnesses including depression, addiction, and anxiety disorders. However, the use of AI in medicine is not without its problems. For example, in the United Kingdom, 63% of the adult population reported that they would not be comfortable with their data being used to improve healthcare which is a significant barrier to the use of AI. These issues could be overcome by using a collaborative approach involving healthcare professionals, AI experts, and patients.
Machine Learning in Neural Pathways
Deep learning is a subset of machine learning that uses many levels of variables and features to predict outcomes. For example, deep learning can be used to create artificial neural networks that may shed light on how psychedelics alter neural pathways.
Using this technique to analyse data from an electroencephalogram time series, researchers discovered the frontal and temporal lobes of the brain are the most affected by Ayahuasca, a traditional Amazonian psychoactive brew. It was determined Ayahuasca increased both closeness centrality (speed neural signals travel to different brain regions) and associativity (strength and closeness of connections) were the main neural networks affected. Interestingly, these measures of the network are negatively impacted during Alzheimer’s disease indicating that Ayahuasca may have therapeutic potential. These results are in line with previous single photon emission computed tomography and magnetic resonance imaging studies, demonstrating the potential of machine learning to accurately identify affected neural pathways in the brain.
Both functional connectivity (FC) and effective connectivity (EC) frameworks show promise for studying lysergic acid diethylamide (LSD)’s neural mechanisms. FC relates to statistical relationships between brain regions, whilst EC focuses on the causal influence brain regions exert over each other. A key concern in using machine learning to analyse psychedelic neural pathways is its accuracy compared to traditional methods. In a study, LSD-induced changes in whole-brain EC were compared to FC through multivariate and machine learning analyses. Significant alterations in FC and EC patterns across brain regions were observed, especially in the angular and inferior frontal gyri. EC closely correlated with LSD's subjective effects. Employing machine learning classifiers trained on either FC or EC data yielded high discrimination accuracy rates between LSD and placebo: 86% and 91%, respectively. Further research is needed for predicting LSD's subjective effects using machine learning models.
Machine Learning Explores Psychedelics and Consciousness
Altered states of consciousness (ASC) are mostly characterised by changes in perception, thought processes, self-awareness, and sensory experiences that can manifest due to psychedelics, hypnosis, dreaming, and meditation. Within the field of psychiatry, there is a growing interest in understanding how ASC manifests when psychedelic drugs are consumed. A better understanding of this may help to minimise adverse experiences or "trips" and offer insights into the mechanism of action of psychedelics.
Machine learning can be used to evaluate whether psychedelic drugs such as psilocybin and LSD, hypnosis, and meditation all cause the same changes in neural networks during ASC. It was demonstrated that psilocybin LSD-induced ASC have similar neural correlates whilst meditation and hypnosis have distinct neural correlates. Both psilocybin and LSD cause visual alterations and have reduced connectivity within the primary visual cortex compared to placebo and meditation. In psilocybin, the “experience of unity” and “insightfulness” correlate with decreased connectivity between regions in the attention related (dorsal attention), higher order cognition (anterior supramarginal gyrus), and language networks. LSD demonstrated that “elementary images” experienced during ASC decreased connectivity in the attention-related (dorsal attention) and the networks involved in awareness of objects, faces, and self.
This study explored the brain activity patterns induced by classic serotonergic psychedelics (LSD and psilocybin) and the dissociative drug ketamine. They found that all three drugs led to reductions in spectral power across different brain regions. Using machine learning, they identified similarities in the effects of LSD and psilocybin in certain frequency bands, particularly theta and alpha oscillations, linked to serotonin 5-HT2A receptor agonism. There were commonalities in the beta band across all three drugs, suggesting connections between serotonergic psychedelics and glutamatergic dissociative compounds in terms of their subjective effects and brain activity.
The results from these studies highlight the need to better understand how psychedelics affect different brain regions and neural networks, especially regarding the subjective ASC experience. These insights could prove invaluable for creating a new era of psychedelics that are efficacious and have minimal adverse events.
Exploring New Horizons: NLP Meets Psychedelics
Natural language processing (NLP) combines linguistics and AI by using computational techniques to analyse natural language. Did you know that NLP is what voice recognition devices such as Google, Siri, and Alexa use to interpret search enquiries? Put simply, NLP teaches computers to speak human. It helps voice recognition devices to identify media, websites, and articles that might be of interest to the user by analysing and grouping commonly used words. More recently, NLP has been investigated as a tool for diagnosing illnesses, predicting responses to treatment, and reducing adverse events.
NLP may be a useful tool to predict treatment response to psychedelics in depression and substance abuse disorder. By employing machine learning alongside speech analytics, researchers were able to achieve an accuracy rate of 85% and a precision rate of 75% in predicting treatment responses to psilocybin among individuals with treatment resistant depression. NLP was also able to differentiate patients with depression from healthy controls with an accuracy of 82.85%. Most importantly when only the patients who machine learning had identified as “responders” were given psilocybin, the overall treatment response increased by 34% compared to the original clinical trial. A second study involving 1,141 individuals who had self-reported quitting or reducing alcohol, cannabis, opioids, or stimulants after a psychedelic experience used machine learning to determine which common descriptions from patient narratives were linked to the long-term reduction or abstinence from each drug. The NLP program was able to distinguish which drug the individual reduced or achieved abstinence from. For example, NLP identified that individuals who “reduced greatly” their alcohol intake used the words “alcohol” and “drink” more in their descriptions. Taken together these two studies suggest that NLP may be a useful tool for predicting which patients are most likely to respond to psychedelics. However, further research is imperative to better understand the exact patient characteristics that predict a response to psychedelic therapy.
NLP has also been investigated as a tool for better understanding the brain regions involved in psychedelic experiences. In a large study involving 6,580 testimonials from individuals who had consumed one or more psychedelics, NLP was used to extract commonly used words and linked them to the neurotransmitter receptors that most likely induced them. Interestingly, despite diverse psychedelic experiences, the researchers could link coherent states of consciousness experiences with receptors and brain regions. The feeling of ego dissolution, a key characteristic of therapeutic potential, was primarily linked to receptor serotonin 5-HT2A. Subjective awareness was most strongly linked to drug affinity at the N-methyl-D-aspartate (NMDA), serotonergic, adrenergic, and sigma-1 receptors in the cortex's primary visual and lateral prefrontal regions and the anterior insula. Vomiting and cramps were mostly linked to the sigma-1 and NMDA receptors in the cortex. These results suggest that machine learning can be an invaluable tool for minimising adverse events by optimising psychedelics to interact with beneficial neurotransmitter receptor combinations.
Machine Learning: A Revolution in Drug Development
Despite the significant strides made in unravelling the mechanism of action of psychedelics, numerous enigmas persist, and the application of machine learning stands poised to provide valuable insights in unravelling these remaining mysteries.
Scientists have used machine learning to generate neural network algorithms which can be given virtual substances. Researchers have been able to generate
N,N-Dimethyltryptamine (DMT) hallucinations using AI to generate deep convolutional neural networks This offers researchers a chance to understand and conceptualise the biological mechanisms that may cause hallucinations and the conscious experience. A better understanding of ASC and hallucinations may help to minimise bad experiences.
Machine learning may also have a role in identifying and classifying the affinity by which drug candidates will bind to their receptors. For example, researchers used 4 different machine learning algorithms in combination with 3D-QSAR to model the affinity of 300 psychedelic substances to bind to the 5-HT2A receptor (5-HT2AR). 75 compounds were evaluated with the field-based and support vector machine models demonstrating high statistical quality and robust predictive potential capability. These models were able to correctly rank the psychedelic substances according to EC50 and the calculated Ki as well as identifying alkaloids already tested for their activity against the 5-HT2AR. The support vector machine model was slightly better at ranking than the field-based model. This suggests that machine learning-based models have the potential to speed up the design of novel therapeutic psychedelics by helping to identify and classify which compounds have the greatest affinity to the target receptor.
Is the Future of AI and Psychedelics Bright?
In summary, the initial use of AI tools such as NLP has provided valuable insights into the mechanism of action of psychedelics and suggests they may be able to predict treatment response. Robust large-scale clinical studies are needed to confirm and expand the patient characteristics demonstrated by AI. The results from the mechanism of action studies using AI must be confirmed both in pre-clinical and clinical settings but may allow for new psychedelic drugs to be synthesised that minimise adverse events. However, it is essential to use these technologies responsibly and in conjunction with rigorous scientific research and ethical guidelines. Additionally, collaboration between AI experts, medical professionals, and researchers in the field of psychedelics is crucial to ensure the safe and effective integration of AI into psychedelic medicine.
References
1. Paul D, Sanap G, Shenoy S, Kalyane D, Kalia K, Tekade RK. Artificial intelligence in drug discovery and development. Drug Discov Today. 2021;26(1):80-93.
2. Amisha, Malik P, Pathania M, Rathaur VK. Overview of artificial intelligence in medicine. Journal of Family Medicine and Primary Care. 2019;8(7):2328.
3. Nichols D, Johnson M, Nichols C. Psychedelics as Medicines: An Emerging New Paradigm. Clin Pharma and Therapeutics. 2017;101(2):209-219.
4. Vayena E, Blasimme A, Cohen IG. Machine learning in medicine: Addressing ethical challenges. PLoS Med. 2018;15(11):e1002689.
5. Gundersen T, Bærøe K. The Future Ethics of Artificial Intelligence in Medicine: Making Sense of Collaborative Models. Sci Eng Ethics. 2022;28(2):17.
6. Davenport T, Kalakota R. The potential for artificial intelligence in healthcare. Future Healthc J. 2019;6(2):94-98.
7. Sanches RF, De Lima Osório F, Dos Santos RG, et al. Antidepressant Effects of a Single Dose of Ayahuasca in Patients With Recurrent Depression: A SPECT Study. Journal of Clinical Psychopharmacology. 2016;36(1):77-81.
8. Alves CL, Cury RG, Roster K, et al. Application of machine learning and complex network measures to an EEG dataset from ayahuasca experiments. PLOS ONE. 2022;17(12):e0277257.
9. Peraza LR, Díaz-Parra A, Kennion O, et al. Structural connectivity centrality changes mark the path toward Alzheimer’s disease. Alzheimers Dement (Amst). 2019;11:98-107.
10. Rubinov M, Sporns O. Complex network measures of brain connectivity: uses and interpretations. Neuroimage. 2010;52(3):1059-1069.
11. de Araujo DB, Ribeiro S, Cecchi GA, et al. Seeing with the eyes shut: neural basis of enhanced imagery following Ayahuasca ingestion. Hum Brain Mapp. 2012;33(11):2550-2560.
12. Bedford P, Hauke DJ, Wang Z, et al. The effect of lysergic acid diethylamide (LSD) on whole-brain functional and effective connectivity. Neuropsychopharmacol. 2023;48(8):1175-1183.
13. Zhang Z, Zhang Z, Ji J, Liu J. Amortization Transformer for Brain Effective Connectivity Estimation from fMRI Data. Brain Sci. 2023;13(7):995.
14. Ort A, Smallridge JW, Sarasso S, et al. TMS-EEG and resting-state EEG applied to altered states of consciousness: oscillations, complexity, and phenomenology. iScience. 2023;26(5):106589.
15. Moujaes FF, Rieser NM, Phillips C, et al. Comparing neural correlates of consciousness: from psychedelics to hypnosis and meditation. Biological Psychiatry: Cognitive Neuroscience and Neuroimaging. Published online July 17, 2023.
16. Pallavicini C, Vilas MG, Villarreal M, et al. Spectral signatures of serotonergic psychedelics and glutamatergic dissociatives. NeuroImage. 2019;200:281-291.
17. Nair IS. Natural Language Processing in Medicine: A Review. International Journal of Engineering Research. 2019;7(05).
18. Carrillo F, Sigman M, Fernández Slezak D, et al. Natural speech algorithm applied to baseline interview data can predict which patients will respond to psilocybin for treatment-resistant depression. Journal of Affective Disorders. 2018;230:84-86.
19. DeSouza DD, Robin J, Gumus M, Yeung A. Natural Language Processing as an Emerging Tool to Detect Late-Life Depression. Front Psychiatry. 2021;12:719125.
20. Ballentine G, Friedman SF, Bzdok D. Trips and neurotransmitters: Discovering principled patterns across 6850 hallucinogenic experiences. Sci Adv. 2022;8(11):eabl6989.
21. Cox DJ, Garcia-Romeu A, Johnson MW. Predicting changes in substance use following psychedelic experiences: natural language processing of psychedelic session narratives. The American Journal of Drug and Alcohol Abuse. 2021;47(4):444-454.
22. Kwan AC, Olson DE, Preller KH, Roth BL. The neural basis of psychedelic action. Nat Neurosci. 2022;25(11):1407-1419.
23. Schartner MM, Timmermann C. Neural network models for DMT-induced visual hallucinations. Neuroscience of Consciousness. 2020;2020(1):niaa024.
24. Floresta G, Abbate V. Machine learning vs. field 3D-QSAR models for serotonin 2A receptor psychoactive substances identification. RSC Advances. 2021;11(24):14587-14595.
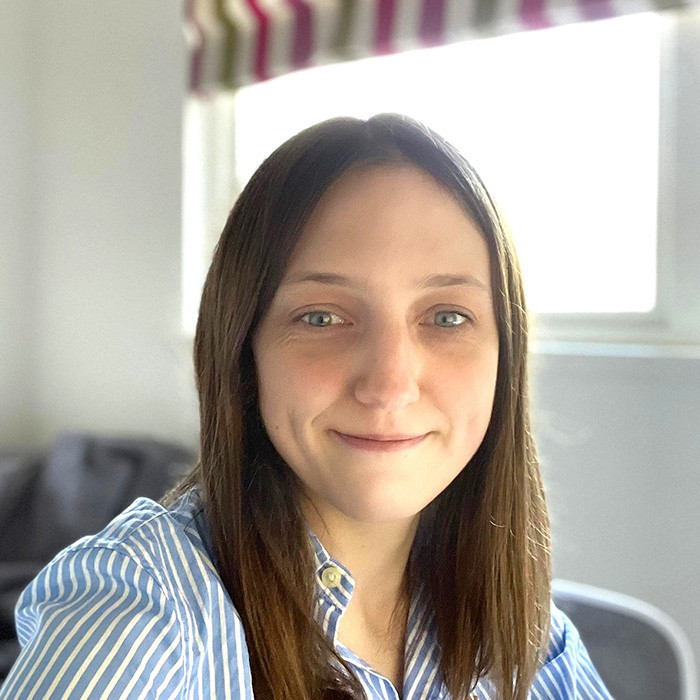
Author
Alice Hamilton
Project Manager and Medical Writer